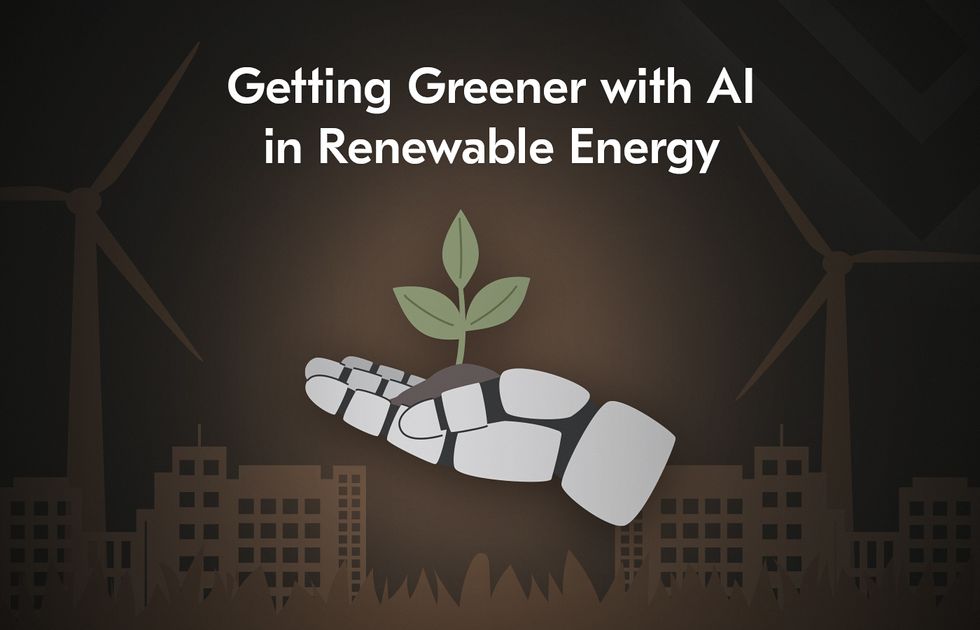
Key Takeaways
- AI is playing a strategic role in improving energy production forecasting, grid management, and sustainability within renewable energy, potentially reducing downtime by 70%.
- The renewable energy market is forecasted to expand from USD 1.34 trillion in 2024 to USD 5.62 trillion by 2033, with AI playing a key role in this expansion.
- AI in renewable energy projects reduces operational expenses by detecting early signs of wear and failure, enabling preventive maintenance and increasing infrastructure lifespan.
- AI has boosted solar energy efficiency by 20% by optimizing panel orientations and tracking sunlight, as seen in Google’s collaboration with DeepMind.
The surging energy demands of cutting-edge approaches are putting immense pressure on global power grids, with AI renewable energy solutions opening new sustainability horizons.
Despite ambitious carbon-neutral pledges, many tech giants see emissions rise as AI-driven workloads expand. But AI itself could be the game-changer. By optimizing solar energy systems, it has the potential to turn a growing challenge into an opportunity.
With solar investments surpassing $500 billion in 2024, AI can help unlock its full potential. It can improve energy forecasting, enhance grid integration, and boost efficiency to deal with inconsistent sunlight, extreme weather, and grid constraints.
Known as one of the best AI developers, Acropolium has been serving the energy industry for 5+ years, breathing automation and predictive models in oil and gas software. Today, we will use the latest insights from our AI/ML consulting experience to tell you how you can reimagine your operation with sustainable energy practices.
The Role of AI in Renewable Energy
AI in renewable energy sector operations is proving valuable in managing systems by analyzing vast amounts of data, forecasting energy production, and enhancing grid stability.
As AI continues to drive advancements across industries — from robotics in logistics to drug development in healthcare — it is also transforming renewable energy. Predictive AI is optimizing solar and wind forecasting, improving grid management, and enhancing energy storage solutions.
These innovations are becoming even more critical as the renewable energy market grows, with projections estimating an increase from USD 1.34 trillion in 2024 to USD 5.62 trillion by 2033.
However, while AI offers significant benefits, it works alongside, rather than replacing, physical infrastructure and regulatory frameworks. Collaborative efforts, transparent data sharing, and strategic integration into national energy policies will be key to ensuring AI’s long-term impact on sustainable energy development.
Benefits of AI in Renewable Energy
In web development, AI is used to promote automation and fast, real-time analysis of essential data. Be it guest analytics in hospitality software or automatic learning plan optimization in education, AI/ML modules greatly improve routine operations. But when it comes to energy, the advantages go far beyond mere automation.
Increased Efficiency and Cost Savings
Handling vast amounts of live and historical data is a challenge for the energy sector. AI in renewable energy makes this process smoother by determining trends, predicting demand, and optimizing energy distribution. Integrating IoT-driven analytics and digital twin technology, it enhances facility management, ensuring a more efficient and reliable energy flow across power plants and grids.
Reducing operational expenses is another key benefit of AI renewable energy solutions. Since AI can detect early signs of wear or failure, it enables preventive maintenance that minimizes downtime. Addressing issues before they escalate extends infrastructure lifespan, ultimately making renewable energy more financially effective for businesses and consumers.
Enhanced Energy Production Forecasting and Grid Stability
The use of AI for green energy initiatives plays an instrumental role in boosting the accuracy of energy production forecasts. This is especially vital for intermittent renewable sources like solar and wind. When analyzing weather data, environmental conditions, and historical energy trends, AI can predict production levels more accurately.
This forecasting approach allows grid operators to balance supply and demand more effectively, ensuring smoother operations and greater grid stability. With AI, energy providers can better anticipate fluctuations, optimize power distribution, and mitigate the risk of grid overloads.
Reduced Operational Risks and Downtime
Maintaining safe operations in high-risk energy environments requires constant monitoring. Renewable energy AI systems focus on workplace safety by analyzing sensor data promptly and monitoring anomalies that could lead to equipment failures or hazardous conditions. Automated alerts and predictive risk assessments then translate to proactive measures, reducing accidents and downtimes by up to 70%.
Better Resource Allocation and Sustainability
Lowering carbon emissions is a priority for the energy industry, and AI green energy protocols are designed to achieve better resource planning and usage. The technology optimizes energy productions and hence helps minimize environmental impact — automating decisions to scale down output during low-demand periods.
At the same time, such systems prioritize clean energy sources and integrate storage solutions for improved efficiency. Together, these efforts create a more sustainable energy ecosystem with an opportunity to increase productivity by 25%.
Acceleration of global renewable energy adoption
Scaling renewable energy solutions requires seamless integration into existing power systems. AI aids in this transition, improving grid management, automating energy trading, and enhancing the performance of microgrids and battery storage.
As AI renewable energy innovations make the sector more reliable and cost-effective, they help attract investment and encourage wider adoption worldwide.
Use Cases and Applications of AI in Renewable Energy
Artificial intelligence is opening up many opportunities in the energy and utilities sector, with many industry leaders adopting it for various tasks. Research and Markets have found that the AI adoption in this field is growing by 24.6% annually as power generation companies steadily embrace AI solutions for higher productivity.
Now, let’s explore the real-world use cases and AI applications in renewable energy that will inspire you to start your own AI journey.
AI for Solar Energy
By leveraging AI-driven analytics, solar energy systems can adjust panel orientations and track sunlight to maximize efficiency. Google’s DeepMind, in collaboration with its own solar farms, has successfully integrated AI to enhance solar power generation, which resulted in a 20% boost in efficiency.
First Solar, a leader in the solar energy sector, has also incorporated AI renewable energy monitoring systems that track voltage fluctuations, panel degradation, and inverter efficiency. With these insights, operators can proactively schedule repairs and balance out their workflows.
AI in Wind Energy
Unlike traditional monitoring methods, AI-powered systems can continuously analyze wind turbine performance, detecting inefficiencies or early signs of mechanical wear. Vestas, one of the world’s leading wind turbine manufacturers, has embraced AI-driven predictive maintenance to optimize turbine performance.
By processing real-time sensor information, renewable energy AI tools can identify subtle vibration patterns or temperature inconsistencies that indicate potential failures.
AI in Hydropower
Hydropower plants rely on water availability, which can fluctuate due to seasonal changes, rainfall patterns, and glacial melt. AI enhances forecasting capabilities by analyzing hydrological and meteorological data to predict water flow more accurately.
Balancing water usage for energy production, agriculture, and ecosystem preservation is a complex challenge. AI-powered models help optimize water release schedules, ensuring an ideal balance between electricity generation and water conservation.
That’s why EDF (Électricité de France) has integrated AI into its hydropower operations — to enhance the sustainability of its water management practices while maintaining stable electricity production.
AI for Energy Grid Optimization
The transition to smart grids has revolutionized electricity distribution by making networks more adaptive and responsive. AI in renewable energy assesses data on power demand, outages, and generation sources to optimize the electricity flow.
Schneider Electric has pioneered AI-powered grid solutions that not only prevent blackouts but also ensure that electricity is efficiently distributed where it is needed most.
AI in Energy Storage and Battery Management
The efficiency of renewable energy systems greatly depends on well-regulated energy storage. AI enhances battery longevity by analyzing charge/discharge cycles, temperature fluctuations, and degradation patterns.
Tesla’s Autobidder, an AI-powered platform, ensures that energy storage assets are used optimally, maximizing battery lifespan while optimizing expenses.
AI for Carbon Footprint Reduction & Sustainability
AI-powered automation has transformed the way businesses and households manage energy use. Smart thermostats, lighting systems, and HVAC controls powered by AI continuously adjust settings to minimize waste. Google’s Nest Thermostat is a prime application of AI in renewable energy, learning user behavior to optimize heating and cooling, leading to substantial resource savings.
Simultaneously, advanced algorithms analyze energy consumption patterns and suggest optimized workflows to reduce carbon footprints. Microsoft’s AI for Earth initiative supports numerous projects aimed at leveraging AI for emission reduction, helping industries transition toward greener energy practices.
Challenges and Solutions for Adopting AI in Renewable Energy
AI has the potential to make a valuable impact on the green energy field, though its broader adoption in the industry comes with its share of challenges. As a seasoned web development vendor, we’ve seen many challenges turn into solutions if aware of the potential failure.
Let’s take a closer look at them and explore solutions that will pave the way for success.
Data Security and Privacy Concerns
Renewable energy AI systems are vulnerable to cyber threats. You can see it from recent incidents like the 2023 cyberattack on the Texas power grid and a Hawaii water utility, with hackers accessing 20+ critical infrastructure entities.
To address these concerns, energy companies can implement sophisticated cybersecurity protocols, including data encryption, multi-factor authentication, and ML-based threat detection. Scheduled and timely security audits and compliance with standards like NIST and IEC frameworks should also be part of your defense against potential cyber threats.
Initial Investment and Infrastructure Requirements
Upgrading energy infrastructure to accommodate AI technology demands heavy investment in sensors, cloud computing, and high-performance systems.
Scalable, modular AI renewable energy solutions can also help reduce upfront expenses, and public-private partnerships allow for cost-sharing and expertise exchange.
The Need for AI-Savvy Talent
A shortage of skilled professionals in artificial intelligence and renewable energy also stands in the way to smooth adoption. To tackle this, it’s worth investing in the development of specialized training programs through collaborations between energy companies and academic institutions.
Additionally, AI-driven automation can ease the burden by taking over repetitive tasks and reducing the reliance on highly specialized personnel. Cross-sector collaboration between tech firms or IT outsourcing providers and energy providers can also facilitate the transfer of knowledge.
Ethical Considerations in AI-driven Energy Decisions
Ensuring that AI systems do not unintentionally discriminate against certain groups or regions. For instance, energy distribution decisions made by AI should not disproportionately favor wealthier areas over underserved communities.
Additionally, there must be clear accountability for decisions made by AI systems. If a green energy AI tool makes an error, it’s important to determine who is responsible and ensure there are mechanisms in place to rectify the situation. Last but not least, ethical concerns arise around how this data is collected, stored, and used, ensuring individuals’ privacy is respected.
One way to address these concerns is by creating explainable AI (XAI) models, which make it easier for stakeholders to understand the logic behind decisions. It’s also important to have regulatory oversight to ensure AI systems align with environmental and social goals.
Why choose Acropolium?
Acropolium is a trusted partner with extensive experience in delivering secure and reliable projects for the energy sector. Putting a strong focus on data protection, we deliver GDPR-compliant software, ensuring that all services meet the highest industry standards.
As an ISO-certified player, we’re committed to quality in any project, from serverless solutions to scalable SaaS architectures and legacy software modernization for advanced energy systems.
Acropolium Case Studies
In the last 5 years, we have provided 6+ comprehensive products and 17+ consultations to our clients. Here’s one of the latest success stories on adopting AI in renewable energy workflows.
Energy Data Analytics Platform
A leading oil and gas client needed an analytical solution to integrate real-time data from drilling rigs, production wells, and reservoir monitoring systems. The goal was to enhance efficiency, optimize resource management, and improve decision-making with real-time insights into performance.
Solution
We developed a scalable energy data analytics platform using Apache Kafka and Apache Spark to integrate live data from SCADA systems, IoT sensors, and production logs into a unified repository. For this AI renewable energy tool, our developers employed machine learning and TensorFlow for predictive maintenance. Key developments included:
- Scalable architecture to handle big chunks of data and support future growth.
- Compliance with industry standards.
- Predictive analytics to reduce downtime and maintenance costs.
- Machine learning for actionable insights in asset management.
- Intuitive UI using React.js and D3.js for data visualization.
- Thorough testing for reliability and ongoing optimizations.
Results
- 15% improvement in production efficiency.
- 20% reduction in equipment downtime.
- 30% decrease in maintenance expenses.
Final Thoughts
The rapid and unstoppable evolution of AI requires specialized skills in machine learning, data science, and computer programming to harness its power. As the energy sector increasingly adopts AI for green energy tasks, keeping up with the new standards is a must to stay competitive.
Acropolium’s dedicated teams have the expertise to address these challenges. We specialize in integrating AI solutions tailored to the oil and gas sector, guaranteeing seamless implementation and optimized performance.
Our team combines deep technical knowledge with industry-specific insights, helping our clients to use the latest technologies effectively within subscription-based cooperation. Contact us to start a new chapter in your business success!