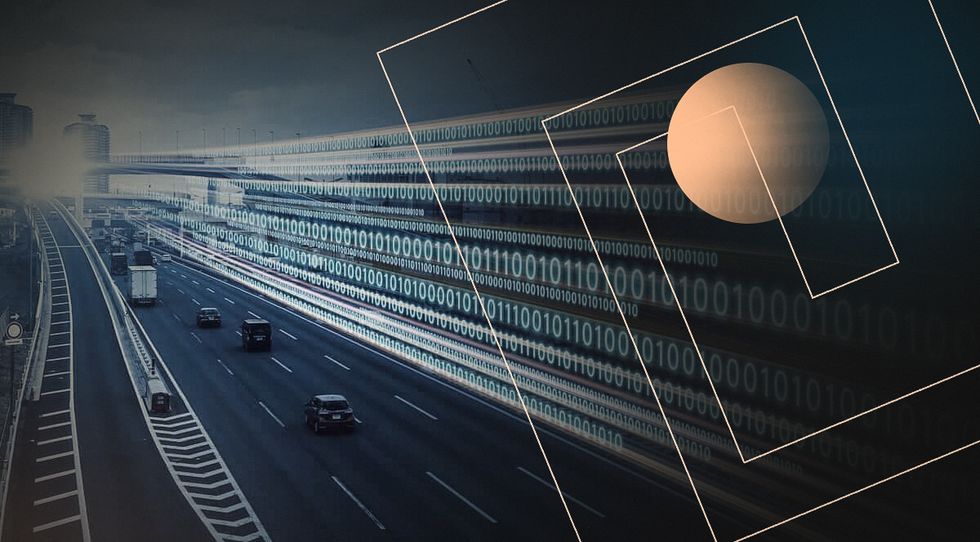
Should you bother with AI when you are simply transporting cargo? Why invest more when you are struggling to cut costs wherever possible?
There’s nothing simple in the logistics business, from supply chain management to hiring truck drivers. AI is not about a driverless fleet at your disposal; it’s about automated processes and cost-efficiency. In this article, we’ll cover the use cases of AI in transportation and how it can help companies that aren’t the size of Amazon. With Acropolium’s help, you’ll discover:
- The real-life benefits of AI in transportation and logistics
- Which companies and startups apply machine learning to their workflow and how they do it
- Where to start if you want to try leveraging AI for your business
“Logistics is a quite old-fashioned industry - they often use paper and feature phones in their routine. AI is something very ambitious and not entirely feasible for transportation business owners. But there’s a big potential for cost-efficiency here, and everything starts from the first step – proper data gathering.”
Oleksii Glib, Founder at Acropolium
The application of machine learning in logistics is not new, but the market shows that only the dominant companies experiment with AI. Decision-makers in the transportation industry named artificial intelligence among the top five technological priorities for their businesses for the next three to five years.
McKinsey states that market leaders who have already adopted AI leverage it within four areas of their business: service operations, product and service development, supply chain management, and marketing and sales. Their key focus fields are asset tracking, connected warehouses, electric vehicles, and process automation.
But many transportation companies are a far cry from an electric vehicle fleet or automated warehouses. Does that mean anyone smaller than Amazon or DHL cannot use AI? A firm no to that. ML algorithms can help you manage your processes better and gain more control over your data. Everything starts with AI-based analytics and advanced decision-making. That’s where you need to look if you strive for cost-efficiency in logistics.
Leveraging AI for transportation: What’s in it for you?
Back in 2017, supply chain businesses lost around $171,340 due to inefficient and cumbersome daily processes. Paper-based routines, operators’ replies to customer inquiries, manual handling of taxes and compliances – all of these bulky tasks can be automated. And if you’re using AI for automation, the efficiency becomes tremendous. How so?
Your transportation company produces volumes of underestimated data, usually poorly stored and partially analyzed. The initial task for any organization wanting to apply ML in logistics is to start gathering information properly, i.e., creating databases. To learn, AI needs gigabytes of information about the various aspects of your business. So, a big data system is a necessity for a transportation AI application.
Using an AI-based solution for data analysis, you will be able to define the optimal vehicle speed for efficient gas and oil consumption, the perfect temperature, load, mileage, etc. You will also improve your management of HR, supply-demand, maintenance, and more. All in all, your decision-making process will be faster and more accurate.
To sum up the business value, here are some key benefits of AI for logistics companies:
- Digitalization of existing processes: you will get rid of paper workflow and reduce human error in your processes
- Proper use of data for decision-making and prediction: all information will be accounted for and applied in a smart way
- Risk management: AI will help you predict market changes and effectively manage your budget
To understand these business advantages better, let’s examine some real-life use cases of artificial intelligence in the transportation industry and showcase some successful examples.
Use cases of artificial intelligence in transportation & logistics
The growing complexity of supply chains, and logistics in general, leaves no room for mistakes and uncertainties. Fast decision-making, instant adaptation to the changing needs of the market, and constant improvement are the only ways to stay competitive. This is why the use of AI in the transportation market is growing.
Artificial intelligence is assisting humans with cumbersome, repetitive, or computationally complex tasks. There are already visible results across the transportation industry, and you can consider them when you start digitalizing your workflows.
Smart supply chain network
Supply chain management is an issue for everyone, from suppliers to retailers. Especially in the circumstances like the COVID-19 pandemic, which shook stable processes that have been working for years. In a time of so much uncertainty and instability, machine learning can make the supply chain less vulnerable to turmoil, but it’s not a stand-alone solution. AI should be part of the DSC – digital supply chain.
DSC leverages IoT, cloud computing, big data, ML and AI, and advanced cybersecurity to ensure full-cycle digital communication. It includes digital conversion of paper documents, demand forecasting and supply correction, prediction of optimal loading schedules and container arrangements, and more. Here’s how it transforms the traditional supply chain:
- Eliminates manual data entry and updates
- Replaces paper systems
- Ensures real-time tracking
- Enables accurate inventory management
- Enhances communication between multiple supply chain members
As a starting point, you can apply AI in the form of an image and language processing system to move all paper data to the computer. It will pull the important information, clean and sort it properly, and store it in an easily accessible form. As the volume of data increases, AI use cases in the supply chain may include analysis and prediction based on the gathered information.
Supply chain management is one of the largest areas where companies start leveraging AI. The reason is that the supply chain is complex and vulnerable to multiple variables. Coyote Logistics (acquired by UPS in 2015) applies machine learning algorithms to predict delays and shipment issues related to traffic and weather conditions. As a result, they are able to create alternative shipment plans and routes as part of risk management.
On a larger scale, the Port of Rotterdam has partnered with Awake.AI to start developing a smart port system based on big data and AI. The examples are numerous since many tech companies offer customized supply chain solutions for businesses of different sizes.
Warehouse management
Organizing warehouses will add to the efficiency of the supply chain. AI can help with several issues here: tracking and navigating products to and inside the warehouses, properly storing them, etc.
The manual work of planning, product alignment, and monitoring is extremely cumbersome and demanding. Employees have to roam the warehouse with a cart full of products and put goods on free shelves, using a scanner to mark their place in the system. And then, they must repeat the process in reverse to find products on the shelves.
The highest level of warehouse automation is robotics, and it isn’t a novelty these days. Amazon first showed interest in robots back in 2012 when it acquired Kiva Systems. Now, it’s leading the warehouse robotics race, with several hundred thousand robots helping out human workers across the entire Amazon warehouse network.
On the other side, startups like Fizyr apply ML in logistics to teach robots to identify, analyze, count, and manipulate warehouse goods.
But the number one priority for any business that wants to stay afloat during the pandemic is not robotics, it’s analytics. The pandemic has been increasing demand for smaller and more diversified product ranges and shorter delivery times, even including same-day delivery. To address these issues, DHL uses the AI-based analytical tool IDEA. It helps to:
- Properly allocate manpower across warehouses
- Optimize picking routes
- Cluster products and orders logically
- Prioritize time-critical orders
With the help of a reliable vendor, you can develop a similar analytical solution and integrate it with your IT infrastructure. Proper data analysis should be prioritized when starting with AI adoption in transportation.
Analytical insights
AI systems for data analysis begin with effective data fusion. The transportation industry produces and deploys gigabytes of data, and artificial intelligence can really help with gathering, storing, and analyzing this data. An AI-based solution like this can fuse and analyze the information from various heterogeneous sources:
- In-vehicle sensors
- Infrastructure IoT
- GPS and vehicle cameras
- Road cameras and radars
- Social media data and more
Efficient data fusion can bring a plethora of other ML applications in logistics, anticipating events, avoiding risks, and aiding in precise decision-making. DHL, for instance, analyzes different sources of internal data and uses AI to predict daily transit times in advance. Also, the DHL AI system analyzes social media posts to identify market changes, potential shortages, supplier issues, and predict supply chain problems. Functionality like this is priceless in times of disruptions and uncertainties.
If the idea of creating your own AI use case in the supply chain seems too ambitious, we recommend starting small. For example, one of Acropolium’s clients decided to build a system to track and analyze the movement of fleet vehicles across various checkpoints. The functionality doesn’t look significant at first, but it brought many valuable insights to the decision-making process. Managers can now identify the most efficient vehicles and drivers, optimize routes, reduce fuel consumption, balance schedules, and more. This is one step toward more complex and advanced fleet management.
Efficient fleet management
Are you thinking of self-driving vehicles? Well, they definitely are part of AI-enabled transportation and logistics. Tesla, Daimler, Volkswagen, and other automotive market leaders are actively working on that, but driverless trucks are still far from mass adoption. Advanced driving assistance, on the other hand, should be considered closely. Cruise control, lane-keeping assistance, automated braking, and distracted driving detection will add to road safety and fewer incidents, repairs, delays, and workforce turnarounds.
Read also: Building Custom Fleet Management Software.
If electric vehicles and advanced ADAS aren’t an option for your fleet at the moment, consider AI solutions for fuel management, compliance control, maintenance prediction, and distracted driving detection. By monitoring engine alerts, pressure, and fuel data in real-time, machine learning algorithms will spot abnormal metrics and provide helpful insights. This information will help reduce downtimes, predict problems, and make repairs more cost-efficient.
Maintenance prediction requires connected IoT devices and an AI-based system for data analysis. One company offering ML solutions for telematics data analysis is Uptake. Logistics and transportation organizations like PepsiCo, Caterpillar, United Road, and others use Uptake’s AI solutions for efficient fleet management.
As for distracted driving, AI successfully spots patterns that indicate drivers’ fatigue, unsafe distances in specific road and weather conditions, and tracks drivers’ time at the wheel. AI techniques used for distracted driving detection include:
- machine and deep learning
- object and face recognition
- computer vision and image processing
Distracted driving is one of the main threats on the roads today, and driver monitoring solutions (DMS) are among the top priorities for authorities and businesses. In 2020, US senators introduced the SAFE Act that prioritizes monitoring drivers’ drowsiness and distractions at the wheel. This means AI systems that can tackle these issues are on the front burner. While there are many possible applications of AI in transportation, you can’t simply decide one day to turn everything upside down and rebuild your processes with AI at the core. How to start smartly and gradually?
Read also: Blockchain technology implementation for transportation & logistics.
How to adopt AI in your logistics business
As with any significant business decision, AI adoption has to meet a specific goal. So the first thing to do is to define the bottlenecks and issues you can tackle with the help of ML in logistics. Analyzing your business processes and setting some long- and short-term KPIs for optimization will draw a realistic picture and help define necessary investments. Note that AI ROI is long-term, so you will probably have to make short-term sacrifices for big gains in the future.
Next, you should assess the technological readiness of your company across three aspects: people, skills, and tools. AI adoption in transportation changes the usual workflow of your personnel. You will have to train existing employees and, most probably, add several new positions to the current staff.
The company’s tech stack needs assessment as well. To properly build an AI-based solution, the vendor or your in-house development team has to explore the possibilities of integration and interoperability with your current systems.
The third stage is deep-diving into your data. Here, you need to consult data scientists and determine what data you need to gather and how to store it properly. Note that this stage is quite time-consuming and technologically complex. To train an AI model, the company has to provide at least several months’ worth of information. It should include as much data as possible about every event, especially non-routine ones – from the driver’s personality to the type of cargo and vehicle sensor data. This approach to data gathering should be followed by all the business fields that will be covered by the AI solution.
After you have a solid database, ML specialists together with data scientists can start training an AI model to do some analytical and predictive tasks. And if we are talking about value – with the basic analytical functionality, you will be able to evaluate the net cost of every transportation within a tenth of a percent. The reason for that is every small piece of information will be accounted for and analyzed.
Read also: How to develop document management system for logistics.
To sum up
The pandemic has shaken the usual flow of things in many industries, and logistics received its hard blow as well. Fast decision-making and the ability to adapt to changes are critical for transportation and logistics companies. AI for transportation & logistics can become a part of the solution if adopted properly.
Paperless operations, greater visibility into processes, the ability to make informed decisions - these are just the basic benefits of applying ML in logistics. With the proper organization and step-by-step implementation, you can turn your supply chain into DSC, automate your warehouses, upgrade your fleet, and significantly improve all of your back-office processes.
Acropolium can help you start smartly and move forward without breaking your infrastructure or disrupting your business too much. Let’s begin with your logistics business audit and see what we can help you with. Acropoliums’s experts are within arm’s length – just give us a call!