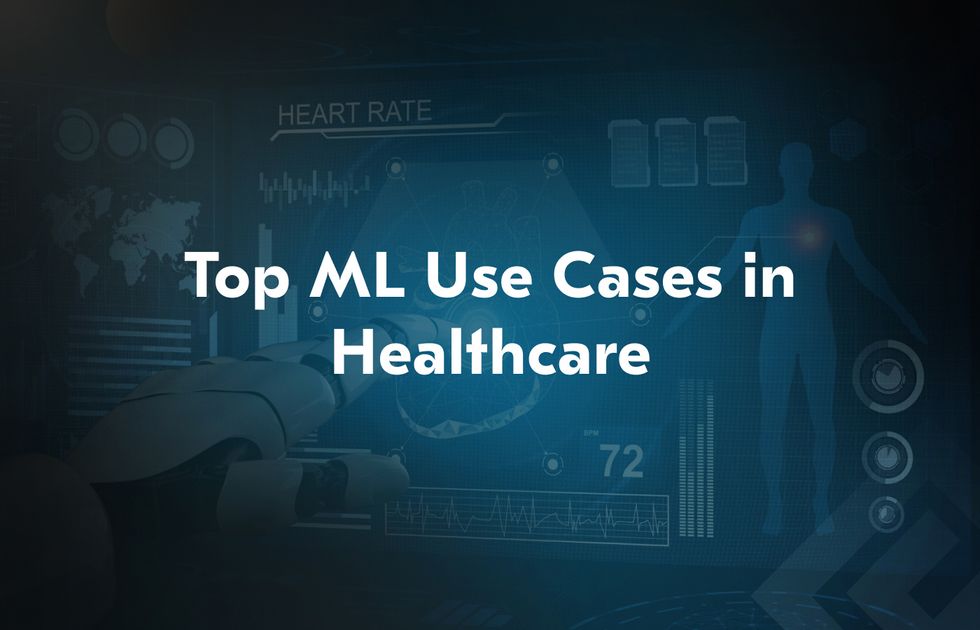
Key Takeaways
- Machine learning in healthcare leverages algorithms to analyze large datasets, enabling better decision-making and personalized care by identifying patterns and continuously learning from data.
- ML algorithms are subsets of AI that help medical providers quickly identify conditions and create accurate treatment plans.
- The global AI healthcare market is projected to grow from $26.69B in 2024 to $613.81B by 2034.
- Healthcare companies allocated 10.5% of their budgets to AI and ML in 2023, up from 5.5% in 2022, with 94% already using these technologies.
- By adopting ML for advanced data processing with Acropolium, our client increased analysis processing accuracy by 40% in their bioscience platform.
Artificial intelligence and machine learning in healthcare have become instrumental in clinical operations, drug discovery, patient care, and medical data management. These algorithms are designed to interact with vast amounts of information, segmenting and allocating insights for better decision-making.
By adopting cutting-edge technologies, medical institutions automate data processing, improve patient experiences, and ultimately bring operational efficiency to the next level. With 15 years of crafting unique healthcare software solutions, we have helped our clients reimagine their medical operations with advanced automated data-first systems.
In this article, we apply our knowledge, insights, and success stories from our 6-year AI/ML consulting and development journey, breaking down the disruptive applications of machine learning in healthcare.
What is ML in Healthcare?
Machine learning in healthcare uses algorithms and statistical models to analyze large medical datasets, enabling better decision-making and personalized care. As a subset of AI, these patterns identify patterns, make predictions, and continuously improve by learning from data.
Different learning types include supervised and unsupervised learning, finding applications in disease classification, and providing personalized treatment recommendations. Due to its wide variety of applications, ML stands among the top healthcare tech trends, along with AI, cloud computing, and robotics.
The pandemic era emphasized the disruptive role of AI and ML in medicine, aiding in the rapid detection and diagnosis of virus strains. AI algorithms analyze patient data to help providers swiftly identify conditions and develop accurate treatment plans. Therefore, the global AI in healthcare market is expected to grow from $26.69B in 2024 to $613.81B by 2034.
Benefits of ML in the Healthcare Industry
The algorithms of machine learning and medicine have been going hand in hand, where technological advancements translate to healthcare industry evolution. This, in turn, has brought tons of growth opportunities for medical businesses while delivering a new level of service to patients.
Morgan Stanley reports that healthcare companies are expected to allocate 10.5% of their budgets to AI and machine learning next year, up from 5.5% in 2022.
Let’s dive deeper into the advantages of machine learning in healthcare operations, both from the medical and business perspectives.
Advanced Disease Detection
ML algorithms are trained on vast datasets of medical images, lab results, and patient records to recognize patterns associated with specific diseases. For example, in radiology, ML models can analyze thousands of X-rays or MRIs to detect subtle anomalies, such as early-stage tumors, that the human eye may miss.
By using ML for healthcare, medical facilities improve their accuracy over time by continually learning from new data, leading to more reliable and earlier diagnoses.
Customized Patient Care
One of the most representational benefits of machine learning in healthcare is that it analyzes patient data, including genetic information, past treatments, and responses to medications. This way, the technology helps to identify patterns that correlate with specific outcomes.
In oncology, ML can predict how different patients with the same cancer type may respond to various treatments based on their unique profiles. This allows doctors to design highly personalized treatment plans, optimizing therapy effectiveness and minimizing side effects.
Risk Prediction and Prevention
ML uses historical patient data to train predictive models that forecast future outcomes, such as the likelihood of disease progression or hospital readmission. Machine learning medical devices analyze variables like vital signs, lab results, and demographic data to predict risks while cooperating with EMR/EHR software.
For instance, in chronic diseases like heart failure, ML models can mark patients at higher risk for complications, allowing clinicians to intervene earlier with preventive measures, reducing hospital admissions, and improving patient care.
Accelerated Drug Development
From a drug development perspective, the impact of machine learning on healthcare is substantive. ML algorithms process massive datasets of chemical compounds, biological interactions, and patient data to identify potential new drugs and predict their effectiveness in treating specific diseases.
They simulate how different compounds interact with biological targets, accelerating the identification of promising drug candidates. This drastically reduces the time spent on trial-and-error experimentation in the lab, leading to faster drug discovery and development.
Streamlined Healthcare Operations
ML analyzes hospital operational data, including patient admission trends, staff schedules, and resource usage, to optimize workflows. Thus, ML models can predict patient inflow based on historical data, allowing hospitals to better manage staffing levels and reduce wait times.
In addition, ML can automate repetitive administrative tasks, such as billing and appointment scheduling, freeing up healthcare providers to focus on patient care. This helps to improve overall efficiency in healthcare delivery and optimize hospital management.
Improved Prescription Accuracy
By analyzing a patient’s medical history, including allergies, previous treatments, and potential drug interactions, ML systems help healthcare providers make more informed decisions. These systems flag potential errors, such as incorrect dosages or dangerous combinations of medications, before they are prescribed.
The use of machine learning in healthcare prescriptions accounts for variations in patient response based on genetic data, allowing for more tailored prescriptions. This reduces the risk of adverse reactions, minimizes human error in prescribing, and leads to better patient outcomes, ensuring safer and more effective treatments.
Use Cases and Applications of ML in Healthcare
While AI comprises many ML applications across industries globally, the modern applications of machine learning in healthcare impress with their effectiveness and wide adoption. In fact, 94% of healthcare companies already utilized AI or ML in 2023. Let’s take a look at some prominent examples.
Predictive Analytics for Patient Outcomes
ML enables predictive analytics by analyzing large datasets to forecast patient outcomes, such as hospital readmission rates or the onset of chronic diseases. For example, NantHealth uses predictive modeling to create patient profiles, identify those at risk of poor health, and offer personalized care plans.
Personalized Medicine
ML healthcare models tailor treatment plans based on individual genetic and medical data. Arizona State University has developed ML models to predict immune responses to new drugs, enhancing personalized care by minimizing adverse drug reactions.
Drug Discovery and Development
By analyzing vast chemical libraries to predict interactions and efficacy, ML accelerates drug discovery. Many pharmaceutical companies like Pfizer and AstraZeneca have used AI to reduce drug development times, which is especially evident during the rapid development of COVID-19 vaccines.
AI-driven models help identify new therapeutic uses for existing drugs, significantly speeding up the research process.
Medical Imaging and Diagnostics
Image recognition capabilities stand among the most impressive machine learning use cases in healthcare. The technology enhances diagnostics by analyzing medical images for early detection of diseases like cancer. Foreseemed uses predictive models to analyze imaging data, identifying early signs of disease progression.
AI’s ability to process vast amounts of imaging data helps improve diagnostic accuracy and reduce human error.
Virtual Health Assistants and Chatbots
AI-driven chatbots provide 24/7 healthcare support, assisting patients with symptom checks and medication reminders. IBM Watson Health utilizes ML to power its virtual assistant, offering personalized advice based on patient interactions.
These tools improve patient engagement while reducing the burden on healthcare professionals.
Remote Patient Monitoring and Telehealth
Machine learning enhances remote patient monitoring and telehealth by processing real-time data from wearables and mobile applications to detect anomalies in patient health. It constantly monitors vital signs and other health metrics, alerting healthcare providers when intervention is needed.
This approach improves chronic disease management, reduces hospital readmissions, and offers proactive care, particularly for elderly and at-risk patients. Biofourmis leverages ML algorithms to analyze patient data from wearables and predict potential health issues before they become critical, offering personalized remote care and reducing hospital visits.
Genomic Research and Analysis
Analysis of genome sequences is one of the most impressive machine learning examples in healthcare. ML helps identify disease-linked mutations, guide personalized medicine, and accelerate drug discovery. The integration of ML helps understand complex biological interactions that would be difficult to detect manually.
Deep Genomics applies the technology to identify genetic mutations associated with various diseases and develop targeted therapies. Their platform uses predictive modeling to understand how genetic changes affect disease progression, supporting personalized treatment options.
Challenges and Solutions in Implementing ML in Healthcare
With all the potential benefits of machine learning in healthcare, it takes a thorough approach, expertise, and adoption readiness to reveal the full power of this technology. Here are the common challenges you should address with an experienced IT outsourcing vendor or web development partner.
Data Privacy and Security
Given that ML relies heavily on patient data, there are significant concerns about the misuse or unauthorized access to sensitive information. Healthcare cybersecurity is integral to ensuring compliance with HIPAA and GDPR regulations.
The solution involves deploying advanced encryption techniques, secure cloud architectures, and anonymization processes to protect patient data. Additionally, companies must maintain compliance by integrating robust governance frameworks and continuously monitoring data security practices.
Data Quality and Accessibility
Another challenge is the quality and accessibility of healthcare data. Clinical data is often fragmented across multiple systems, prone to errors, or inconsistent in format, making it difficult for ML models to produce reliable insights.
The solution to this challenge is standardizing and cleaning datasets before feeding them into your existing software. Also, adopting FHIR (Fast Healthcare Interoperability Resources) standards can help streamline data sharing across both multi-tenant and single-tenant platforms.
Integration with Existing Systems
Implementing ML in medicine requires integrating existing healthcare systems like Electronic Health Records (EHRs) and legacy infrastructure. Not only may it not always be compatible with new technologies, but it can also be costly and time-consuming, creating a significant barrier to ML adoption.
A possible solution is using APIs and middleware to enable smooth cooperation between traditional systems and new ML-powered solutions, allowing for a seamless transition.
Clinician and Patient Trust
Many healthcare professionals are skeptical about AI and ML, fearing that these technologies might replace human judgment or lead to inaccurate diagnoses. Patients may also be hesitant to embrace AI-powered tools, especially if they feel their care is becoming impersonal.
Building trust requires transparency in how ML healthcare models work, rigorous testing to ensure accuracy, and ongoing education for clinicians and patients.
Why choose Acropolium?
Acropolium is a trusted vendor with extensive experience delivering secure, GDPR-compliant software for the healthcare industry. With ISO-certified processes and expertise in serverless solutions, we consistently meet the industry’s highest data protection and reliability standards.
With a clear understanding of the role of machine learning in healthcare, our company continuously explores the technology’s capabilities to breathe success into our client’s projects.
Acropolium Case Studies
From low-code MVPs to comprehensive cloud-based medical systems, we have developed over 23 solutions that have helped our clients from the medical field grow. Here are some of the latest results our clients achieved by embracing machine learning in healthcare with us.
Blockchain-based EHR Software Development
Our client sought to enhance the security and transparency of electronic health records (EHR) using blockchain technology.
Acropolium developed a blockchain-based EHR system, integrating advanced encryption, smart contracts, and a decentralized data storage mechanism:
- A transformative blockchain-based EHR solution was developed under tight deadlines, achieving improved interoperability for seamless integration with existing healthcare systems.
- The enhanced UI/UX boosted adoption rates and user satisfaction while ensuring full compliance with HIPAA regulations.
Cloud-based Bioscience App Development
A bioscience company required a scalable cloud-based solution for big data processing and analysis.
Acropolium created a cloud-based application with AI and ML algorithms designed for processing vast amounts of bioscience data, featuring real-time analytics and a user-friendly interface:
- The project launch time was reduced by 30%, while analysis processing accuracy increased by 40%.
- With the help of well-trained ML healthcare algorithms, the processing time decreased by 38%.
Final Thoughts
Incorporating machine learning in healthcare has become crucial for enhancing clinical operations, drug discovery, patient care, and data management. This technology enables medical institutions to process vast amounts of data more efficiently, improving decision-making and patient experiences.
With 20 years of developing bespoke software for healthcare, e-commerce, hospitality, and many more industries, we strive to transform operations with advanced, data-driven solutions.
Whether you are already starting your AI/ML journey or want to enhance your systems, we offer flexible subscription-based development and consulting services with your budget and goals prioritized.
Now that you know how machine learning is used in healthcare, contact us today — let’s elevate your company’s operations with smart algorithms!