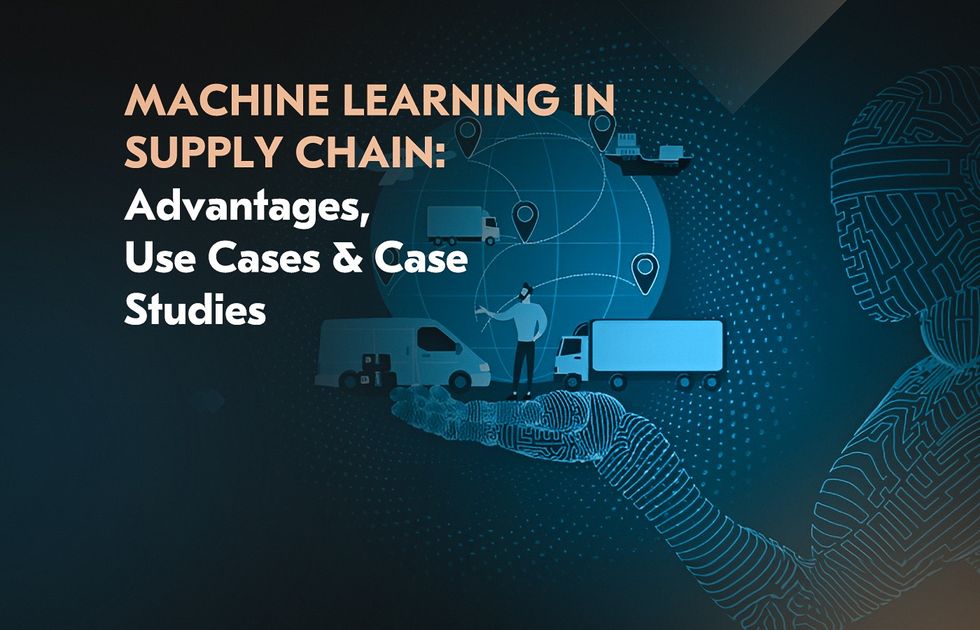
Key Takeaways
- Machine Learning (ML) is a subset of Artificial Intelligence (AI) technology that transforms logistics with accurate data-based predictions.
- According to the Everstream survey, 471 companies reported a reduction in logistics costs after adopting ML-powered automation for data security.
- Implementing machine learning in logistics allows for intelligent analysis of large datasets, enhancing route planning, inventory management, and demand forecasting.
As automation technologies evolve and bring new business opportunities, the logistics industry experiences significant transformations. The role of machine learning in supply chain operations has become vital, with transportation companies striving for optimized deliveries.
With the rise of digitalization, consumer behavior changes for good, and logistics businesses have to adapt to emerging expectations and demands. Accurate, fast, and cheap delivery where goods are tracked to their final destinations is now the new normal.
Serving the transportation sector for 10+ years, Acropolium has delivered 23+ logistics software solutions, implementing machine learning for logistics automation. Today, we will share the advantages of leveraging ML for supply chain operations with use cases and success stories.
Implementing Machine Learning in Logistics: Survey Results
Logistics companies invest in artificial intelligence and machine learning for advanced data analytics to boost efficiency and customer satisfaction. According to Gartner, 50% of supply chain business operations will be powered by AI-based software with progressive analytics features.
Machine-learning-based software allows for automated route building and better demand forecasting, reducing expenses and improving operations. In the Everstream survey, 471 companies report their logistics costs improved after implementing Ml-automation for data security.
Automated Machine Learning Market Size
In its broadest sense, machine learning (ML) is a subset of artificial intelligence (AI) technology. It is used to process and systemize big chunks of data to provide businesses with insights on performance improvement.
The global automated machine-learning market was valued at $6.67 billion in 2025. Forecasted to reach $231.54 billion by 2034, the projected CAGR will grow by 48.3% from 2025 to 2034.
Automation and demand forecasting is where machine learning and supply chain meet to revolutionize transportation business efficiency. Standing among top logistics tech trends, the technology extracts valuable insights from the route, inventory, security, and risk management records.
Utilizing Machine Learning in Logistics Operations: Automation Basics
Machine learning and logistics-oriented artificial intelligence practices focus on streamlining the following activities:
- Demand Forecasting: ML algorithms utilize historical data, market trends, and external variables to enhance demand forecasting, mitigating understocking and overstocking issues.
- Route Optimization: ML optimizes delivery routes in real-time, considering traffic, weather, and delivery windows, reducing fuel costs and delivery times.
- Inventory Management: ML models maintain optimal inventory levels by predicting when and how much stock to order, minimizing carrying costs.
- Predictive Maintenance: ML monitors equipment and vehicles, predicting maintenance needs based on usage patterns while reducing downtime and maintenance costs.
- Warehouse Management: Warehouse optimization machine learning practices help to improve the layout, product placement, and picking strategies, reducing fulfillment times.
- Quality Control: ML identifies defects and anomalies in real-time during manufacturing and shipping, ensuring product quality.
- Last-Mile Delivery: Machine learning optimizes last-mile delivery by considering variables like delivery windows, traffic, and package size, improving customer satisfaction.
- Risk Management: ML assesses supply chain risks by analyzing incident data from various sources, allowing proactive risk mitigation.
- Sustainability: ML in the supply chain helps reduce carbon footprints by optimizing routes and logistics processes, leading to more eco-friendly operations.
Key Advantages of Using Machine Learning in Supply Chain Operations
In transportation, operational efficiency is as dependable on logistics data as on physical assets. From routing performance to inventory and load tracking, every supply chain operator processes vast amounts of data for further growth.
By using machine learning in supply chain management, businesses can reimagine routine tasks with the following benefits:
Improved Efficiency
The popularity of machine learning in the logistics industry is caused by the technology’s ability to foresee potential disruptions. It helps logistics businesses minimize human-factor risks by getting the most out of automatically collected and smartly processed data.
Once the ML-powered software identifies performance patterns, the system will develop algorithms to reduce risks and optimize operations. With live monitored shipments and automatically adjustable routes, companies can reveal the full potential of their assets and fleet.
According to McKinsey, AI-driven forecasting can reduce supply chain errors by 30% to 50%, leading to a 65% decrease in lost sales due to stockouts and a 20% to 50% reduction in inventory levels.
Cost Reduction
Transportation management software powered by real-time data analytics lets logistics businesses plan routes based on traffic and weather conditions. The software will analyze the current traffic, creating the most effective routes and eliminating financial losses.
Real-time shipment tracking can reduce delays by up to 58%, showcasing its impact on cost savings and operational efficiency.
Enhanced Customer Experience
Besides optimizing the business routine, machine learning in supply chain management can dramatically improve customer satisfaction. Such features as real-time parcel tracking, automatic load notifications, and timely delivery strengthen your reputation and enhance customer experience.
This year, 68% of respondents said customer service drives ROI, up 23% from 45% last year.
Better Decision-Making
Machine learning supply chain optimization models imply constant learning from analyzed data. Using mathematical algorithms, it identifies patterns, detects demand signals, and establishes correlations within extensive datasets.
This intelligent system handles large data volumes and continually updates and retrains its model. As a result, logistics operators can turn market-relevant insights into effective planning. According to a 2024 survey by Maersk, supply chain visibility emerged as the top priority among the 15 leading industry trends identified by decision-makers.
Scalability and Adaptability
The more data your logistics software processes automatically, the more adaptive your business gets to the ever-changing market. Machine learning use cases in supply chain management can be as diverse as your company’s scope of tasks.
It’s worth noting that operating with off-the-shelf ML models might only satisfy some of your needs. For larger fleets planning to expand their ML use cases, choosing custom trucking software over off-the-shelf solutions is essential.
AI-powered logistics companies report 20% lower costs, 40% less excess inventory, and a 40% boost in service quality compared to less agile peers due to scalable solutions.
Environmental Impact
ML minimizes waste through accurate demand forecasting, thus reducing warehouse energy consumption and promoting sustainable sourcing.
It also helps track and report carbon emissions, integrates renewable energy, and identifies recycling opportunities. Consequently, using machine learning in logistics contributes to eco-friendly practices throughout the supply chain.
According to a recent Deloitte survey, 71% companies are already addressing sustainability, with another 20% planning to. Meanwhile, 77% report rising demand driven by customer preferences and market trends.
Use Cases of Machine Learning in Supply Chain and Logistics
The number of potential machine learning use cases in logistics varies depending on your scope of operations. Larger businesses might require fleet and inventory management automation, while small operators can only use GPS tracking systems.
The common AI use cases in logistics management usually involve:
- Demand Forecasting
- Smart Inventory Management
- Predictive Maintenance
- Route Optimization
- Warehouse management
- Supplier Planning & Risk Management
- Sustainability in Transportation
Demand Forecasting
Logistics companies can enhance their approaches to forecasting demand in the supply chain using machine learning algorithms and predictive analytics. The AI and ML algorithms unearth patterns that identify demand signals and uncover correlations among variables within big datasets.
These features allow you to understand the current market and customer behaviors better, enabling smart, data-driven decisions. C.H. Robinson — a global third-party logistics provider — has employed machine learning and data analytics to enhance its demand forecasting capabilities. They incorporate real-time data like weather, traffic, and market trends into their ML models for swift responses amid changing demand.
C.H. Robinson has achieved automation across the entire lifecycle of a freight shipment using generative AI, enhancing efficiency and responsiveness in demand forecasting.
Smart Inventory Management
Machine learning inventory management models ensure the supplier products can meet the end user in the right amount and at the expected time. The machine learning algorithms used in supply chain management can predict network-wide demand and recommend efficient actions.
By analyzing current supplies and orders, the system identifies fast- and slow-selling items, reducing shortages and curbing overstock issues. Another excellent example of the successful use of ML can be seen in IBM’s Watson Supply Chain operations. The company leverages ML models to monitor inventory levels and automatically trigger replenishment orders when stock reaches predefined thresholds.
IBM reports that AI inventory management enhances supply chain visibility and automates documentation for physical goods, improving efficiency in inventory tracking.
Predictive Maintenance
While developing custom transportation management software, web development vendors are often asked for vehicle condition tracking features. Though not every AI-powered software supports fleet wearout monitoring, the technology has the potential to aid companies with timely equipment updates.
ML-based sensors monitor critical assets, while predictive models analyze data to forecast maintenance needs.
For instance, DHL, a global leader in logistics and supply chain management, harnesses the power of machine learning to enhance the upkeep of its fleet and equipment. Predictive maintenance alerts are generated, allowing DHL to schedule maintenance proactively and minimize vehicle downtime.
This proactive approach improves efficiency and asset lifespan, reducing operational disruptions and costs.
DHL utilizes noise sensors and machine learning for predictive maintenance of its sorters, which are essential components of automated sortation systems.
Route Optimization
Modern transportation providers can leverage logistics route optimization using machine learning. This process involves identifying the most efficient and fastest driver’s stop sequence while minimizing driving time and distance. Such features also help to monitor and predict traffic patterns impacting delivery times, such as peak hours at logistics hubs. By utilizing ML in the supply chain, businesses can anticipate and proactively mitigate challenges.
For example, UPS — a leading shipping company — has employed machine learning and artificial intelligence to optimize its package delivery operations. The company predicts package volumes at various points in the supply chain. This allows UPS to allocate resources efficiently, reducing delays and improving customer satisfaction.
UPS’s ORION system utilizes machine learning to assess millions of route options daily, optimizing delivery routes and significantly reducing fuel consumption and operational costs.
Warehouse management
Machine learning in warehouse management is applied to automate manual tasks, proactively spot potential issues, and minimize paperwork for warehouse staff. The technology also plays a significant role in programming robots within these warehouses. Furthermore, progressive warehouse management systems involve computer vision that aids in identifying incoming packages and scanning barcodes.
IBM’s Maximo Visual Inspection employs deep learning to automate visual quality inspections in manufacturing, enhancing reliability and efficiency in identifying defects.
Supplier Planning & Risk Management
Transportation agents utilize machine learning in supply chain planning to enhance delivery procedures by meticulously analyzing extensive datasets. The identified data patterns result in advanced algorithms that help to build the balance between demand and supply. This also lowers risks of missed compliance and contrived to better data security.
Amazon employs machine learning algorithms to optimize product recommendations by analyzing user behavior, thereby enhancing customer engagement and sales. At the same time, the company focuses on customer data protection, with IBM’s 2024 report highlighting the increasing importance of AI in cybersecurity, noting a significant rise in the use of AI to detect cyber threats within supply chains.
Sustainability in Transportation
Machine learning enhances the sustainability of logistics operations by enabling smarter, more efficient resource usage. Through intelligent route planning and demand forecasting, ML helps reduce unnecessary mileage, lower emissions, and optimize fuel consumption.
It also supports recycling and waste reduction by tracking material usage and identifying optimization opportunities.
Google’s 2024 Environmental Report highlights that AI-driven logistics planning can cut emissions by up to 30% through route optimization and better fleet utilization.
Implementing Machine Learning in Supply Chain: Challenges and Facts to Consider
Data Quality and Integration
Machine learning relies heavily on data. Since supply chain data can be fragmented, inconsistent, or incomplete, it might pose risks of improper processing. The lack of essential data affects efficient predictions, but this issue can be solved with cloud-computing logistics systems.
Integrating data from multiple sources remains a major challenge, as disparate systems can create data silos that hinder collaboration and analysis. When stored in the cloud, data can be easily accessed from various devices and applications from the logistics management network.
Implementation Costs
Implementing machine learning in logistics can be expensive, including data collection, infrastructure settings, and IT staff-related costs. While ML can be a successful investment, the ROI may not be immediate.
Therefore, companies must plan these investments or address their needs to a verified IT outsourcing vendor for cost-effective implementation.
Address Skill Gaps
The shortage of skilled professionals in machine learning (ML) and data science remains a significant barrier to successful implementation. Companies are facing increasing competition for a limited talent pool, making it difficult to hire or train the necessary experts.
This skills gap slows the progress of ML adoption and its integration into supply chain operations, hindering overall efficiency and growth.
Change Management
Employees may resist adopting new technologies or changing existing processes. Proper change management and training are essential to ensure successful system modernization, yet it requires additional resource investment.
Ethical Considerations
As machine learning becomes more integrated into business practices, ethical concerns surrounding data privacy and algorithmic bias take center stage. The collection and analysis of vast amounts of data must be done responsibly to protect consumer privacy and ensure fairness.
Algorithmic biases can also inadvertently perpetuate inequalities, leading to biased decision-making processes. In response, industry leaders stress the importance of developing responsible AI frameworks to address these challenges, with companies increasingly focusing on creating transparent and accountable AI systems.
Security and Privacy Concerns
Handling sensitive supply chain data requires robust security measures to prevent data breaches and protect customer and business information. When enhancing your supply chain management using machine learning, it’s imperative to operate with the highest cyber-security practices onboard.
Regulatory Compliance
Different regions and industries have varying regulations related to supply chain operations and data handling. Bespoke ML-based software with built-in data handling regulations that fit your requirements is key to keeping your transportation operations compliant.
Why Choose Acropolium?
Acropolium is a certified provider of supply chain management software, delivering comprehensive solutions for the logistics market for 10+ years. From carrier procurement and fleet tracking applications to serverless transportation management platforms, we modernize and build products from scratch.
With 54+ consulting projects and 23+ GDPR-compliant software provided, we realize supply chain business goals while aligning with the budget.
Acropolium Case Studies on Machine Learning
Our solid expertise in the logistics automation market helps businesses broaden their operational horizons. Here are some of our machine learning in supply chain case study examples.
Bioscience Cloud-based Big Data App Development
When cooperating with a targeted proteomics company, our Research and Development was asked to implement biomaterial processing and analysis through AI and ML integration. The client also required back-end visual and technical optimization.
Client goals
- AI & ML Integration
- Back-end optimization
- Data analysis and processing optimization
Challenges
- Our developers had limited communication with an external back-end developer due to their low engagement, which impacted the optimization pace.
- The dedicated team needed to address the issue of low stakeholder engagement during the UAT phase of task workflow. That resulted in chaotic testing and frequent iterations.
Solution & Outcome
- We structured the entire development process for the big data processing app in line with the Scrum methodology.
- By creating a unified framework for managing task lifecycles and daily stand-up meetings, we facilitated seamless communication with external developers.
- Periodic demos let our clients monitor the project’s progress, enabling more effective input on product priorities.
As a result, the client’s processing analysis accuracy increased by 40%, with processing time reduced by 38%. The implemented ML technology and princess optimization helped our partner achieve a 30% reduction in project launch time.
Mobile App Development and Platform Optimization
A large shipping service company sought an IT vendor experienced in supply chain and logistics software. Initially, they requested an MVP mobile app development to track truck driver hours and ensure accurate payment.
Client goals
- Develop a digital solution to solve driver overtracking.
- Provide driver payment assurance in unforeseen situations.
Challenges
- We confronted highly tight deadlines, needing an MVP in just three months to secure investment for future development.
- The forthcoming solution had to address the drivers’ visibility, ensuring transparency over the entire transportation process.
Solutions & Outcome
- Our tech specialists examined the current solution and Android app, identifying critical issues that could impact app scalability and security.
- Prioritizing optimization and bug fixes, we upgraded the existing Android app and built iOS apps within a tight timeframe.
- Acropolium implemented a real-time geolocation tracking feature while implementing a responsive user interface.
- By enabling real-time driver monitoring, drivers can receive automatic payment as their location updates.
Our cooperation resulted in the clients’ 90% increase in security level, with their platform session speed optimized by 28%.
Final Thoughts
As supply chains continue to evolve, machine learning in logistics will be key to staying competitive. From predictive planning to process automation, companies that adopt ML strategically are better positioned to adapt, scale, and lead.
Be it a small, mid-sized, or large transportation business, it takes tons of data to process to stay competitive. Data-driven decisions contribute to intelligent decision-making, cost savings, better route planning, and improved response management.
At Acropolium, we tailor IoT, AI, and ML-based bespoke software solutions to help businesses modernize their logistics. Whether you need a transportation system upgrade or complete product development, we offer a subscription-based pricing model for any goals and budget.
Contact us to reveal the full potential of your logistics!