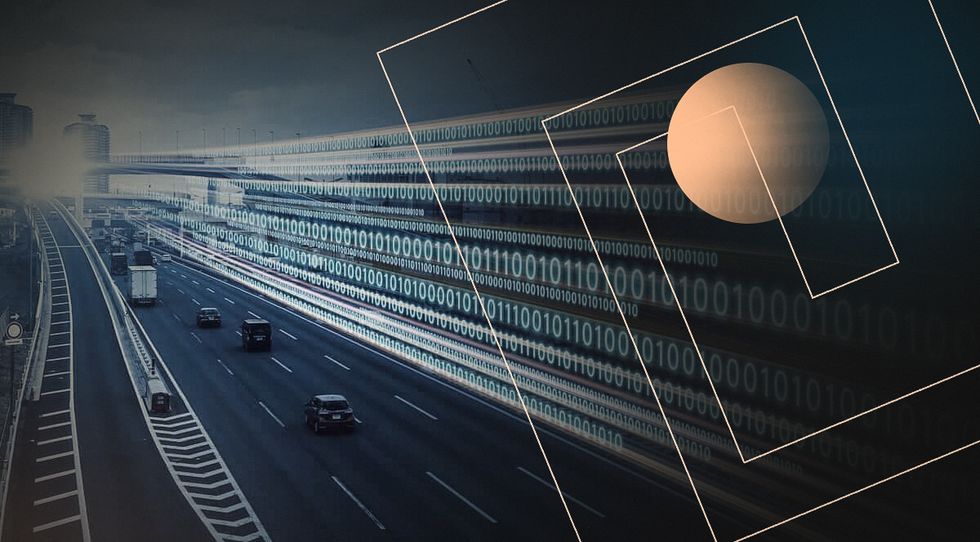
Key Takeaways
- Artificial intelligence use cases in transportation include route optimization, inventory tracking, demand forecasting, and warehouse management.
- Major companies, including Amazon, FedEx, Walmart, DHL, Maersk, and Uber, have adopted AI applications in logistics to enhance efficiency and service.
- The advantages of AI in transportation and logistics include reduced manual work, improved decision-making, cost savings, dynamic pricing, and better customer experiences.
There’s nothing simple in the logistics business, from supply chain management to hiring truck drivers — and companies are looking for smart assistance. That’s why AI in logistics industry reach $17.96 billion in 2024, fueled by advancements in warehouse tech, rising demand in sectors like automotive and healthcare, and increased security needs.
The presence of companies like Microsoft, IBM, and Amazon also accelerates market growth, along with government initiatives and technological innovations in machine learning, natural language processing, and computer vision. Electric vehicle fleets and automated warehouses are not the future but today’s reality.
But how is artificial intelligence used in transportation for companies smaller than Amazon or DHL? Can AI help your startup or mid-sized business optimize processes and reduce costs? Absolutely. In this article, we’ll cover the examples of AI in logistics, its benefits, and the crucial stages of implementation.
The Role of AI in Transportation & Logistics
Let’s start with basic terms: what is AI in transportation?
AI and logistics are all about applying artificial intelligence technologies to streamline logistics operations. By using machine learning, predictive analytics, and automation, providers can handle complex tasks with greater clarity and efficiency, unlocking operational effectiveness.
This transformation is just beginning: in 2025, it’s predicted that 95% of data-driven decisions will be at least partly automated. Yet, surprisingly, only 10% of logistics companies have a strategic artificial intelligence and transportation vision in place today.
AI in the logistics industry reflects this potential, with a rapid growth trajectory from $12.24 billion in 2023 to an estimated $565.82 billion by 2033, marking an impressive CAGR of 46.72%.
This surge is fueled by more than just the cost-saving benefits of AI in logistics. In fact, 27% of supply chain leaders consider gaining or addressing competitive advantage as a key motivation for AI investments, and 9% prioritize it as their main reason for adopting new technologies.
One of the most compelling technology trends is AI-enabled, low-touch planning, which is reshaping supply chain management by reducing manual intervention in decision-making. Advanced supply chain analytics systems facilitate scenario analysis, predict potential disruptions, and autonomously recommend solutions, boosting gross margins by up to 3% and elevating Return on Equity (ROE) by 2 to 4 percentage points.
To illustrate the power of AI and transportation symbiosis, the following infographic from McKinsey highlights the most popular use cases, some of which we will explore in detail in the next sections. From inventory optimization to demand forecasting, these AI applications in transportation are reshaping the way companies operate and create value.
Benefits of AI in Transportation & Logistics
The role of artificial intelligence in logistics process optimization cannot be overestimated. So, let’s get acquainted with the key perks of AI for transportation companies:
Less Manual Work
Integrating artificial intelligence in transportation helps transition from traditional, paper-based systems to automated digital workflows, reducing paperwork and enhancing operational accuracy. Automated processes eliminate manual data entry errors and allow staff to focus on higher-value tasks.
Smart Decision-making and Predictions
Let AI logistics solutions collect, process, and analyze large datasets from various sources like IoT sensors, GPS, and operational data to make informed decisions. At the same time, machine learning algorithms predict trends and optimize routes so you can anticipate needs and efficiently allocate resources.
German logistics leader DHL achieved a 15% boost in operational efficiency with AI-driven predictive analytics.
Risk Management and Reduced Costs
Logistics and AI models forecast market shifts, potential delays, and disruptions, enabling companies to adjust their strategies preemptively. As a result, companies optimize expenditures by choosing cost-effective routes, managing fuel usage, and scheduling maintenance to avoid expensive last-minute fixes.
For example, major logistics companies reported a 27% increase in route efficiency and a 19% decrease in fuel consumption after implementing deep learning methods in their operations.
Dynamic Pricing
Advanced AI models analyze real-time market data and trends to dynamically adjust pricing strategies. This way, you maximize revenue and remain competitive, reacting promptly to changing market conditions.
Better Customer Experience
AI-powered systems allow for real-time tracking and updates, keeping clients informed throughout the shipping process. At the same time, they boost confidence and trust. Such visibility leads to higher satisfaction as clients can plan better around their deliveries.
In 2023, 41% of logistics companies adopted AI-powered chatbots and virtual assistants through NLP, transforming customer service interactions.
Sustainability
What about reduced fuel usage? With optimized delivery routes and schedules, you can easily lower carbon emissions. Ensure sustainable transportation management and compliance with environmental standards for your industry.
For example, North America’s strong focus on sustainability has driven a 21% rise in AI-powered fleet management systems to cut carbon emissions.
To understand these business advantages better, let’s examine some AI in transportation examples.
Use Cases of Artificial Intelligence in Transportation & Logistics
The growing complexity of supply chains, and logistics in general, leaves no room for mistakes and uncertainties. Fast decision-making, instant adaptation to the changing needs of the market, and constant improvement are the only ways to stay competitive. This is why the use of AI for logistics is growing.
Let’s look at the most compelling use cases and successful examples of AI in transportation companies.
Process Automation
Imagine how tedious it can be to perform repetitive tasks such as processing orders, scheduling deliveries, or filling out endless paperwork. Using intelligent algorithms and robotic process automation (RPA), businesses can combine artificial intelligence and logistics to handle these time-consuming operations. This means fewer errors, faster operations, and happier employees who can focus on more important tasks.
Amazon has launched new robotic solutions like Sequoia and Digit to boost safety and efficiency in fulfillment centers. For example, Sequoia, now operating in Houston, speeds up inventory storage by 75% and order processing by 25%, enhancing delivery speed and accuracy.
Real-time Inventory Tracking
Does your inventory tracking feel like a guessing game? Thanks to AI-based technologies like IoT sensors and RFID tags, logistics companies now have an accurate picture of their inventory in real time. No more unexpected shortages or piles of unsold goods gathering dust. In addition, AI predicts when supplies will run out and automatically initiate orders to replenish them.
FedEx Surround enhances supply chain management by providing businesses with exceptional control and visibility over shipments. The solution features an AI-powered dashboard and sensor technology, such as SenseAware ID, for real-time data, enabling quick response to disruptions.
Route Optimization
Want to save on fuel, deliver on time, and reduce emissions? Use transportation and AI duet to analyze traffic data, weather conditions, and real-time road updates to determine the most efficient route. If there is an accident ahead or a sudden road closure, it will instantly reroute drivers to avoid delays. As a result, you reduce expenses and guarantee great customer service.
Walmart’s Route Optimization technology allowed the company to eliminate 30 million miles and 94 million pounds of CO2 emissions by optimizing routes to bypass 110,000 inefficient paths. It also enhances planning for multi-stop journeys, ensures timely deliveries using weather and traffic data, and manages inventory pickups.
Demand Forecasting
One of the biggest challenges for logistics companies is predicting the number of shipments. AI models use historical shipment data, current market trends, and even seasonal changes to predict future shipping volumes. Implement technologies to allocate resources more efficiently, ensuring that there are enough drivers, vehicles, and warehouse space to meet demand.
DHL Express leverages AI-powered logistics software to optimize last-mile delivery, achieving up to 95% accuracy in predicting shipping volumes. This enables precise route planning based on volume and service needs.
Warehouse Management
Organizing warehouses will add to the efficiency of the supply chain. Employees have to roam the warehouse with a cart full of products and put goods on free shelves, using a scanner to mark their place in the system. And then, they must repeat the process in reverse to find products on the shelves. AI in logistics can help with several issues here: tracking and navigating products to and inside the warehouses, properly storing them, etc.
Maersk is deploying an AI-enabled Robotic Shuttle Put Wall System in its new warehouse in East Midlands, UK. This system automates warehouse operations, sorting orders three times faster than manually, enhancing upstream batch inventory picking by up to 33%, and managing 100% of typical SKU assortments.
Analytical Insights
The transportation industry produces and deploys gigabytes of data, and artificial intelligence can really help with its gathering, storing, and analyzing. It can use sources like in-vehicle sensors, infrastructure IoT devices, GPS, vehicle and road cameras, radars, and even social media. Efficient data fusion can bring a plethora of other applications of AI in logistics, anticipating events, avoiding risks, and aiding in precise decision-making.
Uber Freight is launching Insights AI, a generative AI tool that analyzes transportation dynamics and identifies key drivers for better decision-making. Using 45 data points from millions of shipments, it provides proactive insights to optimize transportation outcomes, answering critical questions about performance and trends in shippers’ networks.
How to Adopt AI in Your Logistics Business
1. Identify Goals and Bottlenecks
Start by clearly identifying what you aim to achieve with AI in logistics and transportation — streamlining processes, reducing costs, improving delivery times, or enhancing customer experience. Use KPIs tailored to each goal to ensure a clear benchmark for success.
Examine your logistics operations to find recurring problems or inefficiencies. This could range from delivery delays, high operational expenses, or poor inventory management. Defining these challenges helps in choosing the right AI tools for your needs.
2. Assess Technological Readiness
AI in transportation industry changes the usual workflow of the staff. Evaluate your team’s current skill set to see if they have experience with AI and big data analysis. Plan for upskilling existing staff or hiring data engineers and ML developers.
Is your web app architecture strong enough to process large data volumes and support AI operations? Explore the possibilities of integration and interoperability with your current systems.
3. Data Preparation
The third stage is deep-diving into your data. Collaborate with data scientists to determine its type and quality. They will collect your historical data, operational logs, driver and vehicle information, and sensor data from logistics equipment.
Implement or upgrade data storage systems to manage the large volumes of structured and unstructured data necessary for training AI in the transportation industry. Ensure data is clean, accurate, and organized to avoid unreliable outcomes.
4. Model Training and Testing
Work with machine learning specialists to start training models based on prepared data. Depending on your goals, these models can handle tasks like predictive analysis, route optimization, and demand forecasting.
Test the AI models using a controlled environment to see how they handle real-world data. This phase may require adjusting algorithms or retraining the model based on performance feedback.
Why Choose Acropolium?
Looking for a trusted IT outsourcing partner from Ukraine? Acropolium has 10+ years of creating and modernizing SaaS solutions for transportation and logistics, as well as AI-powered software for healthcare, hospitality, and other industries. We are proficient in advanced technologies, such as IoT, GPS and GIS (Geographic Information System), ML, and blockchain.
Our top development agency builds GDPR-compliant software, following ISO-certified processes to ensure high security and quality standards. A dedicated team also specializes in creating serverless solutions, providing scalable supply chain management, route and load planning, fleet management, shipping & freight forwarding, inventory & warehouse management, and document generation systems.
But what business results can you achieve with the help of AI-powered SaaS software? Let’s consider specific cases as an example.
SaaS Route Planning Tool for Fleet Management Optimization
A transportation provider needed a scalable solution for growing delivery volumes. Acropolium developed SaaS route planning software integrated with ML algorithms for real-time traffic analysis and automated route optimization. This included fleet management features for driver performance tracking and vehicle location oversight.
The company saw a 15% profit increase from reduced fuel consumption and costs, while customer retention rose by 25% due to better delivery accuracy. Logistics efficiency improved by 25% with optimized coordination, real-time tracking, and resource management.
Supply Chain Data Analytics Software
Another of our clients was struggling to manage its complex network of warehouses, distribution centers, and transportation hubs. To overcome this, our specialists built an advanced supply chain analytics platform that consolidated fragmented data from various sources, including ERP systems, IoT sensors, and GPS devices. Also, we applied predictive analytics for better demand forecast and inventory management.
The company experienced a 20% reduction in system downtime, a 27% increase in operational efficiency, and a 15% decrease in inventory costs. Customer retention also rose by 22%, thanks to better service delivery and personalized engagement.
Final Thoughts
The logistics industry is changing rapidly due to digital transformation, the growth of eCommerce, and sustainable app development trends. Today, artificial intelligence and machine learning are being used to optimize supply chains, speed up operations, and improve decision-making.
Acropolium, with its experience in the development of advanced custom software, helps transport and logistics companies navigate these changes. We can help you start smartly and move forward without breaking your infrastructure or disrupting your business too much — on a subscription basis. Contact our reputable agency to conduct an audit of your business and learn the answer to the question: “How is AI used in transportation more efficiently?”